(suggested citation: Mishra, D. and Pal, J. (2020) Indian journalists’ influence on Twitter https://joyojeet.people.si.umich.edu/indian-journalists-influence-on-twitter/)
Overview
The goal of this work has been to map the networks of influence on Indian social media. We took the top 110 journalists based on the number of other journalists who follow them from our sample of 1899 journalists to create a seed set of key journalists in India. A list of the journalists who were key influencers in terms of “following” behaviour is available here.
This list shows us a landscape of journalists with important voices, but also highlights several key problems with the sample, a significant leaning towards English-language journalists. We did a follow-up to seek an alternate means of understanding “influence” to seek out which journalists were retweeted by key politicians. The results are available here, and this list has a far higher number of journalists whose primary work is not in English.
In line with other ways of understanding “influence” we mapped key journalists’ social media behavior and following to seek their footprint in the months of January and February 2020. By footprint, we measured their median retweet rate (as opposed to arithmetic mean which would be biased by viral tweets), as well as the total number of retweets to see how much a journalist gets mentioned.
Findings
As we see in figure 1, a small number of journalists have a hugely outsized impact in terms of the net retweets their messages get – Rana Ayyub, Arfa Khanum, Faye D’Souza and Abhisar Sharma get fairly significant engagement to their messaging. However, some less obvious accounts are also important. Vinod Jose, Somesh Jha, Nitin Sethi, Arvind Gunasekar, Supriya Sharma and Milind Khandekar all have significant footprint despite relatively much smaller following.
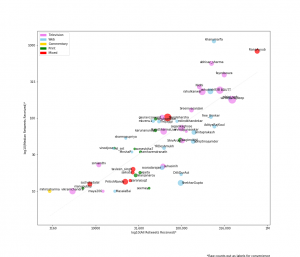
We see a dominance of television and digital journalists (which are often made up of people who were formerly in some traditional format) as compared to those who are largely print journalists. We excluded from our sample commentators who were widely retweeted. The categorization of journalists into these was somewhat arbitrary, and we classified as “mixed” journalists whose work typically appeared across formats.
The reasons for being retweeted can be varied, and it is one, albeit incomplete measure of online influence. Retweets can be as a result of mastering short-form messaging, having a niche following, or being part of a topic or position that typically trends. Thus some of the most retweeted journalists are also routinely among the most trolled and harassed. For instance, two commentators who have mastered the short form, particularly using a mix of are Akash Banerjee and Dhruv Rathee, both score higher in median retweets than almost all the journalists in this list.
The frequency of tweeting also impacts the amount of retweets one gets. For instance, accounts that tweet fairly frequently (not including retweets), such as Shivam Vij, Suhasini Haider, and Seema Chisti get relatively fewer median retweets. The most prolific tweeter was the Wire’s Rohini Singh who tweeted and engaged in conversation with people on Twitter almost twice as much as any other journalist on this list.
We also looked at two other metrics. One, whose messages were more “political” for which we used a crude metric of the use of politicians’ handles, as opposed to other methods such as a “bag of words model” or hasthtag mapping to do a more complete topical model. Ashutosh and Rajat Sharma are among journalists who mention politicians in a very high proportion of their tweets.
Finally, we see that the use of URLs is another important metric. Journalists who use a high proportion of links are typically providing a link to a story that has more information, thus acts as a plug for both the story and the organization. Shekhar Gupta, Ashutosh, Sidharth Varadarajan, Nikhil Wagle, and Paronjoy Guha Thakurta all include URLs, typically links to reports from their respective organizations, in a majority of their tweets.
Methodology
Using Twitter’s public API we sourced their tweets(~110k) for the months of January and February 2020. We removed all accounts that tweeted less than 300 times in this period, to focus on the most active social media accounts. We only count retweets of original tweets, not of retweets of other persons’ tweets. More methodological details are in previous articles in this series here.
Table 1: Key statistics for various journalists and their online influence
Rank (median RTs) | ID | Total Tweets
(Jan-Feb) |
% Tweets that are Retweets
|
Number of politicians mentioned | Followers (start of period) | % Tweets with URLs | Sum RTs | Median RTs |
1 | khanumarfa | 637 | 58% | 75 | 355688 | 19% | 237399 | 1133 |
2 | RanaAyyub | 1278 | 26% | 30 | 876194 | 29% | 756470 | 826 |
3 | abhisar_sharma | 2267 | 90% | 337 | 713813 | 15% | 222694 | 562 |
4 | fayedsouza | 481 | 7% | 25 | 896593 | 59% | 313034 | 391.5 |
5 | Nidhi | 1780 | 60% | 168 | 609467 | 14% | 160440 | 268 |
6 | BDUTT | 823 | 15% | 148 | 7068088 | 29% | 278118 | 242 |
7 | ashutosh83B | 544 | 15% | 666 | 1997404 | 75% | 215951 | 233.5 |
8 | rahulkanwal | 336 | 55% | 33 | 4428992 | 13% | 173344 | 230.5 |
9 | rohini_sgh | 5923 | 49% | 771 | 280800 | 12% | 364277 | 186.5 |
10 | sardesairajdeep | 778 | 13% | 134 | 8871256 | 41% | 387289 | 179 |
11 | SreenivasanJain | 573 | 28% | 24 | 565280 | 32% | 130002 | 129 |
12 | free_thinker | 2128 | 49% | 66 | 216735 | 33% | 218752 | 104 |
13 | bhogleharsha | 643 | 34% | 2 | 8440876 | 14% | 68572 | 102.5 |
13 | gauravcsawant | 768 | 21% | 99 | 1422889 | 22% | 59278 | 102.5 |
15 | rahulpandita | 686 | 49% | 20 | 97629 | 15% | 49756 | 98 |
16 | mkvenu1 | 594 | 34% | 68 | 143452 | 32% | 46606 | 91.5 |
17 | milindkhandekar | 623 | 24% | 36 | 100282 | 39% | 88212 | 91 |
18 | TheJaggi | 1824 | 89% | 194 | 160585 | 15% | 54391 | 90 |
19 | AdityaRajKaul | 2191 | 30% | 144 | 252494 | 18% | 240469 | 77 |
20 | sagarikaghose | 601 | 30% | 100 | 4117016 | 44% | 99292 | 73.5 |
21 | karunanundy | 1039 | 34% | 106 | 123919 | 30% | 49407 | 68 |
22 | RajatSharmaLive | 369 | 4% | 212 | 3849460 | 13% | 61715 | 66 |
22 | arvindgunasekar | 728 | 32% | 37 | 53243 | 31% | 113224 | 66 |
24 | smitaprakash | 1462 | 30% | 42 | 751123 | 17% | 138850 | 64 |
25 | sharmasupriya | 486 | 42% | 2 | 45625 | 51% | 22350 | 53 |
26 | waglenikhil | 1167 | 31% | 83 | 840941 | 63% | 96982 | 50 |
27 | ShivAroor | 1305 | 20% | 39 | 898206 | 23% | 101971 | 49 |
28 | abhijitmajumder | 988 | 11% | 41 | 539513 | 33% | 135946 | 48 |
29 | vinodjose | 936 | 89% | 30 | 21337 | 11% | 16478 | 39 |
29 | YRDeshmukh | 1968 | 45% | 125 | 175796 | 14% | 62280 | 39 |
31 | nit_set | 1570 | 86% | 120 | 28656 | 11% | 16110 | 38.5 |
32 | someshjha7 | 1296 | 60% | 108 | 20809 | 17% | 28369 | 38 |
33 | MnshaP | 1411 | 63% | 53 | 40088 | 20% | 26342 | 34.5 |
33 | iamnarendranath | 748 | 41% | 64 | 51670 | 13% | 33088 | 34.5 |
35 | soniandtv | 337 | 67% | 10 | 834328 | 31% | 10859 | 23 |
36 | suhasinih | 2581 | 66% | 122 | 1282035 | 24% | 60680 | 22 |
37 | svaradarajan | 1323 | 35% | 98 | 544143 | 65% | 59129 | 21 |
38 | tavleen_singh | 419 | 44% | 18 | 615776 | 48% | 27200 | 20 |
39 | vijaita | 1508 | 46% | 95 | 83482 | 25% | 32773 | 18 |
39 | saikatd | 1563 | 65% | 75 | 162027 | 18% | 26975 | 18 |
41 | DilliDurAst | 4806 | 68% | 337 | 97314 | 22% | 98581 | 17 |
42 | nilanjanaroy | 1224 | 34% | 98 | 215888 | 33% | 29130 | 16.5 |
43 | paranjoygt | 1415 | 41% | 79 | 80931 | 61% | 25177 | 14 |
44 | PritishNandy | 1294 | 57% | 146 | 1953861 | 10% | 22035 | 13.5 |
45 | ShekharGupta | 1669 | 9% | 44 | 2215505 | 85% | 97369 | 13 |
46 | suchetadalal | 928 | 52% | 124 | 689273 | 29% | 8538 | 12.5 |
47 | seemay | 2511 | 64% | 119 | 30289 | 29% | 41764 | 11 |
47 | manupubby | 793 | 51% | 44 | 43399 | 25% | 7105 | 11 |
50 | vikramchandra | 867 | 73% | 73 | 2993692 | 21% | 5361 | 10 |
50 | mihirssharma | 448 | 70% | 15 | 155097 | 21% | 2906 | 10 |
50 | maya206 | 727 | 58% | 63 | 68479 | 32% | 12145 | 10 |
50 | MasalaBai | 1473 | 38% | 71 | 72275 | 14% | 16676 | 10 |