Asmit Singh, Lalitha Kameswari, Jivitesh Jain, Tushar Mohan, Aravind Narayanan, Dipanwita Guhathakurta, Ponnurangam Kumaraguru, Joyojeet Pal
We examined the use of Twitter by 10426 politicians from Uttar Pradesh, across all levels of seniority, midway into the phases of polling in the Uttar Pradesh elections of 2022, starting January 1, 2022 and up until March 1, 2022. We summarize here our key findings by examining four key questions.
How does Twitter relate to Urbanization and Literacy?
To address the first question, we examined the number of urban residents by location, and levels of literacy, and find there is a statistically significant relationship between both the number (and percentage) or urban residents and the likelihood that politicians in that area use Twitter, as well as with the level of literacy and the likelihood of tweeting. We removed Lucknow district from the equations we ran, since it is an outlier being the capital where most parties have a number of listed post holders.
We see some variation — for instance, the district with the highest urban populations – Ghaziabad, adjoining Delhi, and Kanpur, both have fewer politicians online than Varanasi, the Prime Minister’s constituency, which has had a lot of mid- to lower-level politicians go online, and Allahabad / Prayagraj, which is a politically important district in the state, and home to several key politicians from across parties.
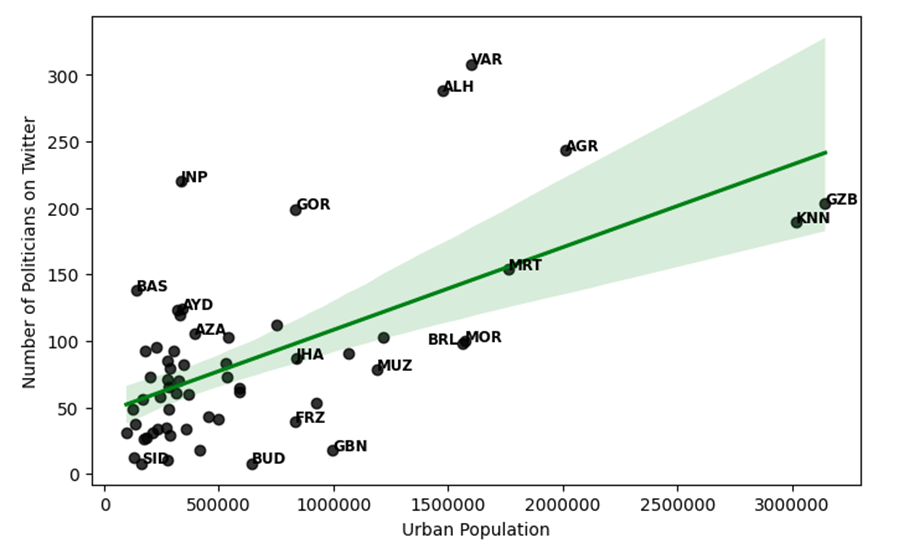
Figure 1: Number of urban residents in a district correlated with the number of politicians from that district active on Twitter
Taking the number of politicians on Twitter as the dependent variable and the urban population of the district as the independent variable,and found a strong correlation between the 2 with an R value of 0.61. The coefficient 𝛽1 was found to be significantly different from 0 (p<0.05). (See Appendix Table 1)
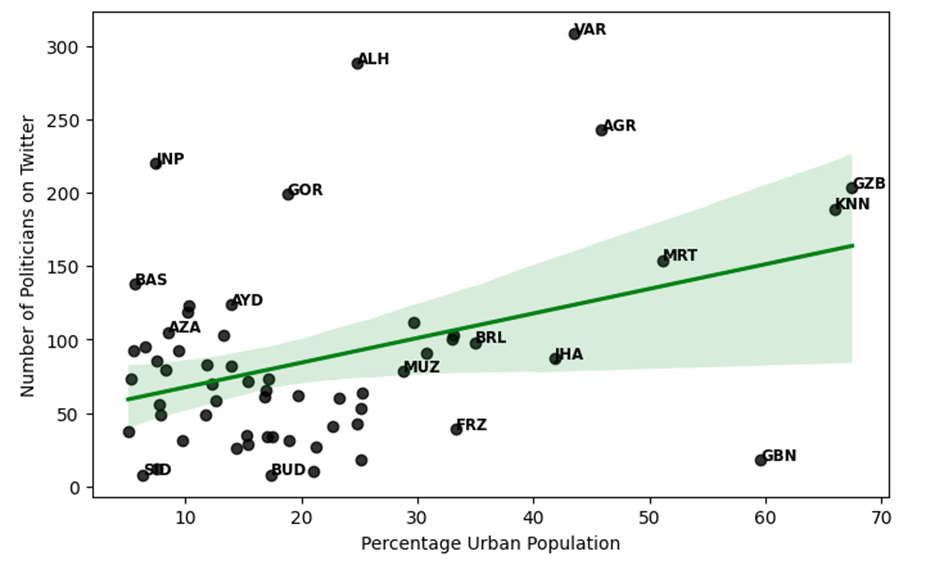
Figure 2: Percentage of urban residents in a district correlated with the number of politicians from that district active on Twitter
When we ran the same regression to correlate the percentage of urban residents to the number of politicians active on Twitter, again we found a strong statistically significant (p<0.05) relationship between the two variables with an R value of 0.37. There are outliers. On the lower end, we see that Gautam Buddha Nagar (Noida) has a smaller share of politicians active online (partly because of the cross-listing with Delhi) whereas Gorakhpur, an overwhelming majority rural district has a high number of politicians active on Twitter. This again can be attributed to CM Yogi Adityanath, who contests from the district. Please see Appendix 2 for details.
We see the same relationship between the number of literate persons in a district and the number of politicians. The relationship here is the strongest, statistically significant (p<0.05) with an R value of 0.78. Figure 3 below shows us a visualization of the districts. Again, we see that Varanasi is an outlier, for the reasons outlined above. Please see Appendix 3 for details.

Figure 3: Number of literate residents in a district correlated with the number of politicians from that district active on Twitter
As a sanity check we ran the same regression on the share of literate persons in a district, we again find a statistically significant relationship (p<0.05) with a strong relationship of rate of literacy with the n of politicians on Twitter at an R value of 0.78. Please see Appendix 4 for details.
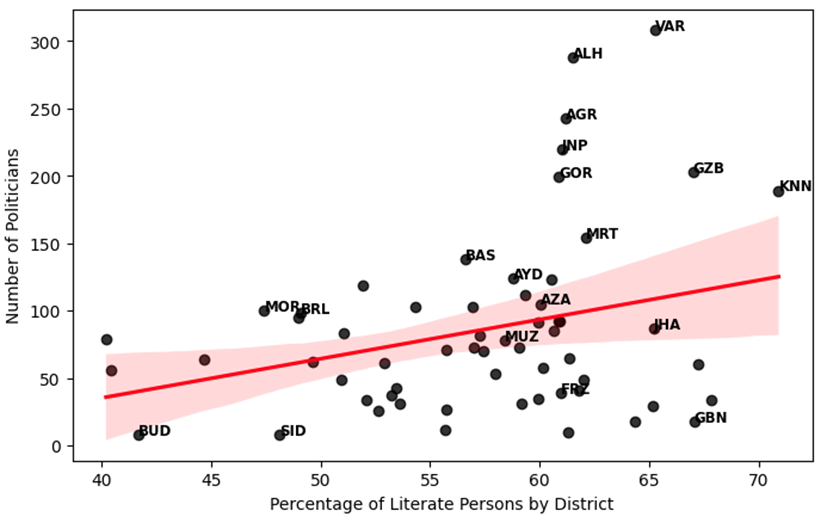
Figure 4: Percentage of literate residents in a district correlated with the number of politicians from that district active on Twitter
Where’s the Action?
To detail these findings, we detail the data by district. Using a crude method of looking at the number of active politicians and the rate at which they get retweeted, we examined the districts in which one or another party had a clear Twitter dominance. We restricted our analysis to those districts that had 50 or more active politicians online. The districts which had a clear BJP Twitter advantage (where the local networks of politicians on Twitter were larger, at least two of the top three accounts belonged to that party, and the party was more active on social media) were Ghaziabad, Amethi, Mathura, Barabanki, Kaushambi, Gorakhpur. The districts where the Samajwadi Party had a clear advantage were Banda, Fatehpur, Etawah, Prayagraj (Allahabad), and Azamgarh. The districts where the Twitter battles were evenly matched across multiple parties were Kannauj, Ballia, Lucknow, Sultanpur.
District(min. 40 active politicians) | Code | SPPoliticians | BJP Politicians | Other Party Politicians | SP per- politician median Tweet count | BJP per- politician median Tweet count | Other party per- politician median Tweet count |
Agra | AGR | 49 | 125 | 52 | 49 | 111 | 179.5 |
Aligarh | ALG | 14 | 51 | 26 | 150 | 82 | 111 |
Prayagraj | ALH | 123 | 84 | 59 | 100 | 53.5 | 142 |
Amethi | AMT | 15 | 91 | 53 | 58 | 78 | 85 |
Ayodhya | AYD | 28 | 74 | 14 | 28.5 | 165 | 162 |
Azamgarh | AZA | 53 | 15 | 25 | 68 | 141 | 329 |
Bahraich | BAH | 40 | 23 | 7 | 59 | 102 | 3 |
Ballia | BAL | 26 | 33 | 25 | 165.5 | 115 | 131 |
Balrampur | BRP | 12 | 14 | 24 | 36 | 108 | 212 |
Banda | BAN | 25 | 27 | 16 | 105 | 65 | 84 |
Barabanki | BAR | 26 | 61 | 19 | 40 | 78 | 63 |
Bareilly | BRL | 33 | 32 | 19 | 34 | 68 | 157 |
Basti | BAS | 21 | 76 | 28 | 17 | 120 | 241.5 |
Bhadohi | BDH | 12 | 32 | 10 | 91 | 119.5 | 235 |
Chandauli | CHD | 14 | 29 | 9 | 21 | 117 | 26 |
Deoria | DEO | 33 | 63 | 17 | 53 | 97 | 69 |
Etawah | ETW | 37 | 15 | 6 | 80 | 94 | 159 |
Fatehpur | FTH | 34 | 19 | 7 | 77.5 | 130 | 128 |
Ghaziabad | GZB | 23 | 121 | 47 | 118 | 211 | 122 |
Ghazipur | GZP | 25 | 40 | 15 | 77 | 83.5 | 68 |
Gonda | GON | 20 | 47 | 17 | 52 | 120 | 109 |
Gorakhpur | GOR | 34 | 124 | 27 | 88.5 | 130.5 | 159 |
Hapur | HAP | 7 | 31 | 27 | 44 | 205 | 199 |
Hardoi | HAR | 21 | 53 | 12 | 59 | 58 | 98.5 |
Hathras | HAT | 4 | 17 | 10 | 320.5 | 145 | 86 |
Jaunpur | JNP | 66 | 100 | 43 | 90 | 84.5 | 145 |
Jhansi | JHA | 26 | 28 | 26 | 48.5 | 177.5 | 93.5 |
Kannauj | KAN | 26 | 26 | 8 | 109 | 102 | 97.5 |
Kanpur Dehat | KND | 15 | 16 | 6 | 29 | 288.5 | 283 |
Kanpur Nagar | KNN | 58 | 81 | 24 | 63 | 136 | 74.5 |
Kaushambi | KAU | 7 | 30 | 7 | 50 | 138 | 150 |
Lucknow | LCK | 442 | 406 | 226 | 113.5 | 158 | 147.5 |
Mathura | MAT | 12 | 53 | 38 | 19.5 | 89 | 102.5 |
Meerut | MRT | 21 | 77 | 38 | 96 | 137 | 197 |
Mirzapur | MIR | 17 | 39 | 20 | 56 | 41 | 109.5 |
Moradabad | MOR | 27 | 48 | 14 | 32 | 80.5 | 92 |
Muzaffarnagar | MUZ | 13 | 26 | 30 | 56 | 213 | 144.5 |
Pratapgarh | PGH | 22 | 44 | 14 | 96.5 | 75 | 19 |
Rampur | RAM | 11 | 29 | 14 | 28 | 103 | 80 |
Saharanpur | SAH | 13 | 42 | 20 | 60 | 137.5 | 185 |
Shahjahanpur | SHJ | 15 | 23 | 12 | 26 | 85 | 127.5 |
Sitapur | SIT | 24 | 28 | 14 | 28 | 123 | 144.5 |
Sonbhadra | SON | 10 | 37 | 12 | 34.5 | 230 | 500.5 |
Sultanpur | SUL | 24 | 30 | 16 | 30 | 81 | 101.5 |
Unnao | UNN | 16 | 32 | 8 | 59.5 | 34.5 | 148.5 |
Varanasi | VAR | 87 | 137 | 74 | 69 | 111 | 162.5 |
These data points may be skewed by the effectiveness of IT cells or small cores of supporters. It is also true that the INC’s IT cell is fairly well organized and networked, but most on-the-ground analyses seem to discount its likelihood of winning seats proportionate to its social media footprint. However, these data underline the massive dominance that the BJP has over other parties on Twitter.
District Code | Top most followed active state politician handle from the district | Party | 2nd most followed active state politician handle from the district | Party | 3rd most followed active state politician handle from the district | Party |
JAL | Rashmipal_86 | SP | bpsvermaoffice | BJP | mohammad_ubaid_ | INC |
LAL | ArchanaPatelAD | APNA | inckuldeepnsui | INC | nitinpanthltp | BJP |
HAM | DipakSurjeet | SP | Aryan_Vaishnav9 | INC | arunodayINC | INC |
KSH | RadheshyaSingh | SP | Sunny10113594 | BJP | Bjp4shubhamsahi | BJP |
ETA | ashishyadavmla | SP | visheshYSP | SP | AsRathoreBJP | BJP |
CHK | BhaironMishra | BJP | KishanGupta_INC | INC | AtuleshanandM | BJP |
FRZ | DrChandrasenBJP | BJP | Prashan25958727 | SP | RohitYadav5500 | SP |
GBN | satender_awana | BJP | Sandeepsharmak7 | BJP | AshuChhawri1717 | BJP |
BAH | NitishRastogi01 | BJP | Shivafauji | BJP | nishanktripath1 | BJP |
MAH | yadavyogi1 | SP | ManojA2Y | SP | Neerajbuddh_ASP | BSP |
KAS | inderveshverma | BJP | ABVPVikas89 | BJP | TejendraLodhi | BJP |
AUR | AAPlogical | AAP | manju27bjp | BJP | Narendralodh73 | BJP |
BRL | sachink71656735 | BJP | JabirAkhtarKhan | AIMIM | DrPramendr | BJP |
AMR | SatyadeoPawar | BJP | VishalVermaJi00 | BJP | kstanwarmp | BJP |
HAP | anandktrbjp | BJP | ramesh4Churu | BJP | SarswatPrafful | BJP |
KAN | dineshsngyadav | SP | poonamy49617468 | SP | socialistarchna | SP |
BIJ | Ashokkatariya9 | BJP | Digvija27100456 | BJP | BRBJPBIJNOR | BJP |
KND | Up32Wale | BJP | iABHIKSHA | BJP | AnkitSinghZ | BJP |
HAT | SharadBJPoffice | BJP | islamkhan919 | SP | RamAvtarValmiki | INC |
BAS | pal_jagdambika | BJP | Shweta4Nation | BJP | BajrangbaliMa | BJP |
BAN | Shubham41489417 | BJP | HariNMishra | BJP | AAPkaRamGupta | AAP |
BRP | Dr_Mannan_ | AIMIM | HKhan_293 | INC | Vibhuonline | INC |
AZA | amitydvsp | SP | IamShaikhAdil | AIMIM | NishantRaii | SP |
BDH | ashutoshgyanpur | BJP | AkashDubeyBJP | BJP | ratnakarbjp | BJP |
HAR | jeetusp | SP | bjpsaurabh12 | BJP | PrachiPretesh | BJP |
FTH | MahipalMahla | BJP | anjuydv | SP | YunusSamajwadi | SP |
GON | gopalkagarwal | BJP | KVSinghMPGonda | BJP | BNSinghbobby | BJP |
ALG | SatishGautamBJP | BJP | kuldeepgaurBjp | BJP | LakhendraMeena | INC |
AMT | DeepakSinghINC | INC | BJP4Amethi | BJP | dtripathi264 | BJP |
MAI | spsunilyadav | SP | LalitMYOfficial | SP | Samajwadi_mpi | SP |
CHD | DrMNPandeyMP | BJP | mlasadhanasingh | BJP | IshanMilki | SP |
JHA | Socialist_arun | SP | AnuraagJhansi | BJP | pradeepjain52 | INC |
GZP | Virendra_mla | SP | manvendrabjp | BJP | rajeshysp | SP |
GOR | myogiadityanath | BJP | AgrawalRMD | BJP | kamleshpassi67 | BJP |
KAU | BJPVinodSonkar | BJP | BabluGargBjp | BJP | prashant3k1 | BJP |
BAR | priyankaMP_BBK | BJP | upendrasinghMP | BJP | PushpendraBjpKm | BJP |
DEO | manishagautamak | BSP | msirsiwal | INC | BirendraYdvSP | SP |
AMB | mpriteshpandey | BSP | ankitchandelbjp | BJP | 12tiwari25 | AAP |
AGR | BjpRajora | BJP | AbhilashaBJP | BJP | Rajkumarchahar9 | BJP |
MAI | yadavakhilesh | SP | SubratPathak12 | BJP | RanjeetYadavSP1 | SP |
BAL | sparvindgiri | SP | upendratiwari_ | BJP | virendramastmp | BJP |
AYD | pawanpandeysp | SP | LalluSinghBJP | BJP | PanditSamarjee2 | SP |
KNN | sdPachauri1 | BJP | ptopmishra | SP | manojku72692521 | SP |
BAG | Phulesavitribai | KBMP | AnilTomarBjp | BJP | RLD_Baghpat | RLD |
ALH | Vndnason | AAP | yogita_singh13 | SP | RakshaMantri | SP |
ETW | socialistaditya | SP | kuwarprashant | SP | Samajwadi_AY | SP |
JNP | YRBSamajwadi | SP | OsamaShaikhIND | INC | Hb_o12 | BJP |
GZB | Gen_VKSingh | BJP | druditatyagi | BJP | preeti_chobey | SP |
LCK | CMOfficeUP | BJP | BJP4UP | BJP | samajwadiparty | SP |
Who does the party think gets the votes, Modi or Yogi?
We examined the number of tweets that referred to Narendra Modi and Yogi Adityanath to get a sense of who the party expects will win the elections. The results show that while Yogi Adityanath had a higher footprint early in the campaign in January, that got replaced by Modi by February, to the point where Modi is now consistently the more featured of the two leaders in terms of both the engagement by party politicians and workers, and equally importantly, by the party’s main social media handle.

Figure 5: Line graph of the number of times Narendra Modi and Yogi Adityanath are mentioned in tweets by BJP politicians through all UP districts during the study time period. We find six events corresponding to the peaks (calculated over two day interval):
1. Jan 6, Modi peaks for 150 crore milestone for covid vaccinations
2. Jan 9 Yogi peaks for communal 80 vs 20 tweet about BJP’s win in UP
3. Jan 14 Yogi peaks for Magh mela, announcing contest from Gorakhpur
4. Jan 26 Modi peaks for Republic day and UP state formation day address
5. Feb 1 Modi peaks for virtual rallies
6. Feb 9 Modi peaks for televised ANI interview
7. Feb 23 Modi peaks for public gatherings in Prayagraj, Amethi, Hardoi etc
We also checked for this pattern in the main handle for the BJP in the state – @bjp4UP. Here, we see that Modi was virtually missing from the discourse in much of January, and re-emerged only in February, and since mid-February has in fact been more important in the party’s outreach than the Chief Minister.

Figure 6: Visualization of the number of mentions weekly of @narendramodi and @myogiadityanath by @BJP4UP handle in between Dec 31 and March 3.
We visualized the words that appeared most commonly in the tweets of that included @narendramodi and @myogiadityanath during the study period, excluding common tweets and we found that the co-occurring tweets tell a very interesting story about the way BJP leaders and the party establishment sees the two figures in its outreach. To arrive at these, we merged synonyms (thus सपा is merged with समाजवादी), we remove common words articles, common verbs (thus में, श्री, और, है etc) as well as words that are purely informational (चुनाव, जनसभा, मतदान, सत्ता etc) and words that have no political consequence in the context of the tweets (प्रदेश, लोग etc), and are left with terms that are either explicitly part of the political discourse, or are about locations of relevance.

Figure 7: Wordcloud visualization of co-occurring words in tweets including @myogiadityanath – size of the words proportionate to the number of mentions
We can see that the differences between the two leaders and the tweets mentioning them are significant. While there are common themes (Kisan, Garib, Vikas) we can see that there are two striking differences. First there are differences in the locations that the two sets of tweets mention Saharanpur, Kashi (his constituency), the Yogi-centric tweets mention Gorakhpur (his constituency), Ayodhya, Muzaffarnagar.
Second, we see that the Modi-centric tweets take aim at the familial continuity of power (through the use of “parivarvaad”) – aimed at both Akhilesh and Priyanka, but primarily at the former. While both leaders refer to farmers, they are a lot more central to Modi-centric messaging. Likewise while “Corona” and “Vaccine” also on tweets about both leaders, the centrality of vaccine success, as well as the touting of marquee initiatives like Atmanirbhar are more seen in Modi-centric messaging.
Finally, the Yogi-centric messaging has one overarching theme — that of sectarianism. We see a number of terms either referring to Samajwadi party in veiled references to rioters, terrorists and criminals through the use of terms like अपराधियों, आतंकियों, माफिया, दंगाइयों, सुरक्षा but in addition, the Yogi-centric tweets are also more likely to use words such as भगवान, रामभक्तों, शिलान्यास, हिंदू, मंदिर etc.

Figure 8: Wordcloud visualization of co-occurring words in tweets including @narendramodi – size of the words proportionate to the number of mentions
Of all the major contenders, probably the least active on social media is the Bahujan Samaj Party, and a number of their candidates are not active on Twitter. In general, Akhilesh Yadav is far more engaged than the two key other opposition leaders – Priyanka Gandhi and Mayawati, however, Priyanka Gandhi has gradually closed the gap with Akhilesh.
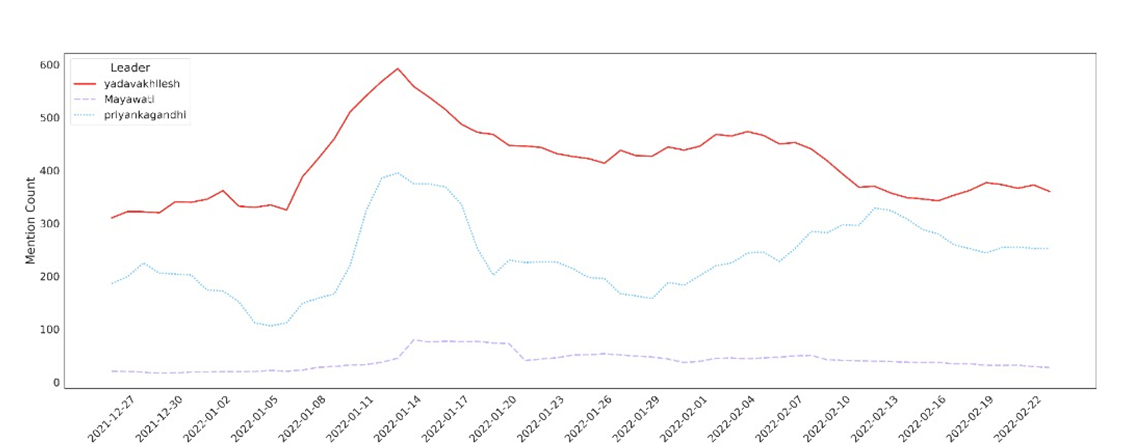
Appendix / Statistical Details
Slope | 6.20e-05 |
Intercept | 46.16 |
R value (Pearson’s correlation) | 0.611 |
R Squared | 0.374 |
P Value | 2.089e-07 |
Standard Error | 1.054e-05 |
Table 1. Regression table for Politicians on Twitter = 𝛽0 + 𝛽1 * Urban Population + 𝜀𝑖
Slope | 1.67 |
Intercept | 50.72 |
R value (Pearson’s correlation) | 0.37 |
R Squared | 0.14 |
P Value | 0.003 |
Standard Error | 0.54 |
Table 2. Regression table for Politicians on Twitter = 𝛽0 + 𝛽1 * Percentage Urban Population + 𝜀𝑖
Slope | 7.24e-05 |
Intercept | -32.78 |
R value (Pearson’s correlation) | 0.78 |
R Squared | 0.61 |
P Value | 1.19e-13 |
Standard Error | 7.52e-06 |
Table 3. Regression table for Politicians on Twitter = 𝛽0 + 𝛽1 * Literate Population + 𝜀𝑖
Slope | 2.51 |
Intercept | -84.66 |
R value (Pearson’s correlation) | 0.07 |
R Squared | 0.61 |
P Value | 0.03 |
Standard Error | 1.17 |
Table 4. Regression table for Politicians on Twitter = 𝛽0 + 𝛽1 * Percentage Literate Population + 𝜀𝑖
Methodology for comparing the mentions of Narendra Modi and Yogi Adityanath by the BJP politicians
We collected all the tweets of BJP politicians over a period of around two months (from 31st Jan 2022 to 3rd Mar 2022) and counted the number of mentions which Narendra Modi and Yogi Adityanath got. We plotted this data keeping a rolling 2-day average to capture the trends in increase and decrease of mentions and correlated the peaks with relevant events.